At the beginning of the 19th century, the industrial revolution changed many manual jobs, and that led to a better quality of life.
It also resulted in the short-term loss of many jobs. Since then there has been a recurring fear that technological change will lead to mass unemployment.
Although, the kind of Artificial Intelligence and Machine Learning revolution that the world has considered to be, will be quite different from the Industrial Revolution.
AI has been around for so many years now with its own highs and lows in terms of public opinion. Although, in the most recent years, it has spiked up to the forefront due to the increasing power of hardware as well as infrastructure.
There has been much interest by the GAFA i.e., Google, Apple, Facebook, and Amazon companies that added fire to the growth of AI and ML in the last few years.
Even though there might be much enthusiasm and excitement about AI technologies, their application in software testing is still a growing bud.
Questions are also being raised if AI will be the ‘doom’ to the testers’ community and will AI be responsible to perform all security in the future?
How AI Integration Benefits Testers?
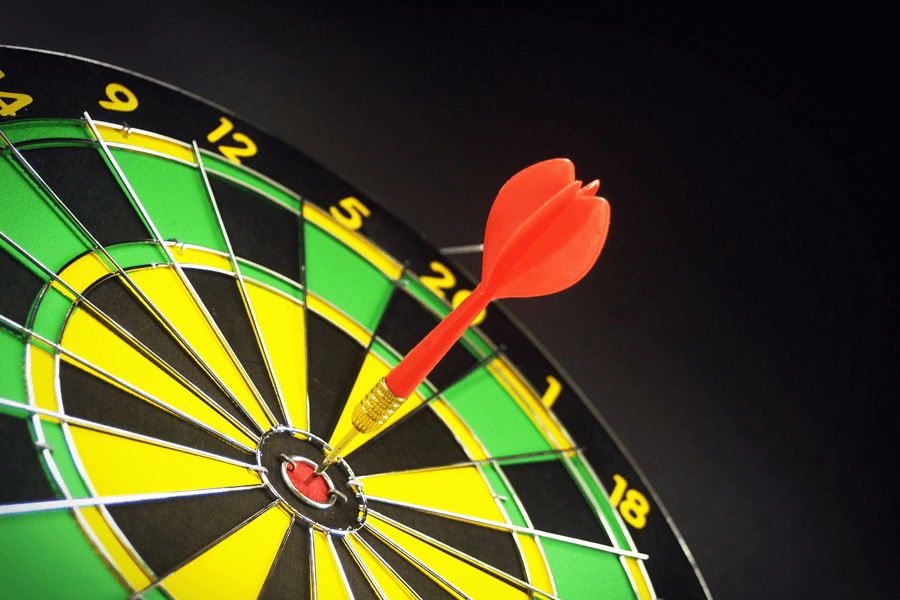
1. Improved Accuracy
Conventional testing still needs manpower for the source as well as data analysis. However, this is no secret that even the veteran QA experts are bound to make mistakes, which is completely fine.
The testers quite often lose their focus on software quality assurance and miss out on some crucial defects due to comprehensive data.
Before the project stakeholders get an opportunity to realize it, users get ahead of the curve and spot out the bugs.
Generally, such situations can be quite detrimental to the brand rapport as well as product positioning.
This is where the AI and ML technologies come into the picture. They teach mechanisms to know about source analysis so that they apply that system in the future. Thus AI testers offer improved results.
Leveraging AI technologies for data analysis will eliminate the human error occurrence, reduce runtime of a test, and detect potential defects.
The QA team, due to this, is not overwhelmed with huge data silos to manage.
2. New Portfolio for a Tester
The future artificial intelligence screening of 2021 will effectively handle the entire screening process. QA engineers will develop new and unique skill sets; as it is going to be the future of an immense volume of screening.
Operating with AI will seek multiple talents in number-crunching optimization, algorithmic analysis, neuro-linguistic programming, business intelligence, and AI testing.
The professionals of the World Quality Report predict the shortage of highly qualified experts, via practicing mature AI. Therefore, the firms need to upskill their QA staff to face this challenge.
The general and latest issue of the report recommends three potential roles of QA experts:
a. AI Test Professionals
Including the testing nuances, they also need to develop machine learning potentials about math patterns and also have expertise in natural language execution systems.
b. Data Scientists
Being a quality assurance team expert, analysts sort data, utilize statistics, and develop predictive analysis to create the required patterns for artificial intelligence-based quality assurance strategy.
It is forecasted that QA professionals will transform into test automation experts. They will work as an invigilator and teach AI to perform an array of screenings. AI can take control of nearly 70% of the recurring testing space.
Experts will be in demand to handle the test results and focus on the remaining screening handling the user interface tests, for instance.
Additionally, test managers will remain accountable for tooling, workflow process, and environmental situations.
While AI could be a great help in executing recurring screenings, still a QA expert will determine the progress, account for the test plan, and monitor the QA strategy and the goals.
c. AI QA Strategists
The team has to manage the artificial intelligence role in the business functioning. With the great knowledge of data patterns, number optimization, and robotics methods, they find various ways to incorporate AI QA standards into the entire business process.
d. Predictive Analysis
The market demand in the IT field never ceases to grow, the businesses require to find a way to predict customers` needs and stay ahead of the curve from the competitors.
This is a difficult job for predictive analytics for software testing companies. AI and machine learning may assist in quick customer data analysis to simplify their preferences in newer products and features.
How Machine Learning can contribute to Testing?
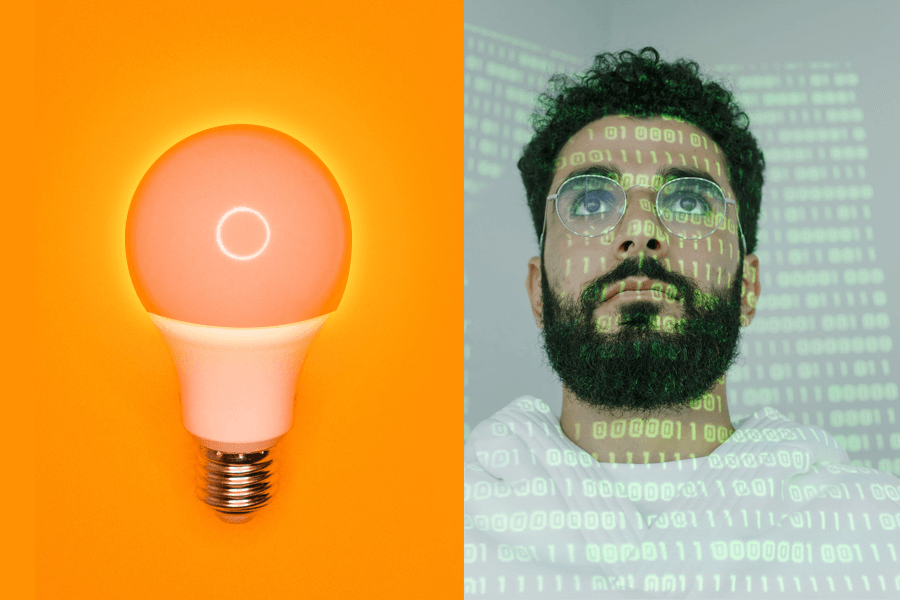
ML technology works on pattern-identification. It examines the pattern of information and recognizes predictive algorithms.
It changes the automation testing system, as machine learning testing can be done without any user interface. Most of the automated QA is not a front-end-based system.
1. UI Tests
The ML bots can be useful while dealing with the UX. Most of today’s apps have the same system in functionality, design, or even interface.
Say, for instance, you can quite easily detect a product filter, shopping cart, and also a payment page in an eCommerce site.
You can train the bots for a specific software portion to handle more test cases compared to regression testing.
Image-driven testing, using the visual verification systems is a catchy system that ML identifies. QA engineers can build an easy ML test that would automatically detect visual flaws in software.
2. Strategy-orientation
Generally, QA experts run a complete test system just due to small changes along with the script. The use of machine learning tools can let them define the minimum tests required to test the potential of the code modifications. It tests vulnerable software parts and the latest test areas.
3. APIs
Machine learning testing initiatives can help to organize an efficient diagnosis of the API layer. The equation takes control of the analysis of test systems, while a tester isn’t stuck to make multiple APIs.
Challenges on the Way
Nonetheless, AI appears to be a benchmark for the QA trends, it is not quite simple to make it that huge and real. It faces a range of hindrances blocking the acceptability of AI in testing.
1. Silos of Data
To run tests on the haphazard data added human resources, as well as funding, is needed.
Today, companies can hardly maintain huge data evaluation and can`t go with the utilization of cognitive and robotics platforms.
2. Artificial Intelligence Integration
For the moment, companies are unable to identify the business areas to utilize artificial intelligence technologies for improvement.
Additionally, the majority of the QA firms can`t seem to make a way to use AI systems. The teams require professionalism first to utilize AI in their business functioning.
3. Lack of Artificial Intelligence knowledge
As said already, intelligent testing widens testers` skills and proficiency. It is just a matter of time when this gap will be bridged.
Although, the demand for qualified engineers for Artificial Intelligence testing in 2020 will drastically increase and more companies will try to deploy “intelligent QA” in their mainstream functioning.
AI-based Test Automation Systems
This is a fact till now that the invention of Artificial Intelligence is unavoidable. However, whatever the speculations might be, we think that the time is not ripe yet for AI to take over the complete testing equation.
In this day and age, business and QA experts talk much about the “third wave of testing automation” as a unique AI automation testing system.
Let’s find out what are they:
1. Applitools
This visual testing system is yet another Artificial Intelligence product. This system helps a lot to highlight the predictable layout of an app.
It provides a visual comparison system to detect and report any discrimination found in the UI of an app.
This system is great for performing instant automated tests and detecting hundreds or thousands of interface similarities in seconds.
2. Eggplant AI
This system, with the help of intelligent algorithms, runs through the software. It finds out the defects and resolves the glitches regarding updated data connection.
It automates any test automation system and provides a graphical analysis of test coverage as well as results.
3. Appvance
The tool provides an in-depth evaluation of software using ML and generates “app blueprints” patterns utilizing cognitive development.
The blueprints brag to produce many test cases in a fraction of time. Besides Artificial Intelligence, Appvance incorporates Test Designer, a record-and-playback aspect.
It integrates data-based tests, screenshot evaluation, along with AJAX or DOM auto capturing.
4. Test.ai.
It recognizes the elements and screens of software and deploys an app to drive out test cases.
It adjusts to any alteration of a component and also identifies the pieces that need manual rectifications.
5. Testsigma
It is an AI-based algorithm for consistent automated testing. This tool uses a natural language testing method to write high-quality automated tests.
Testsigma recognizes the suitable test cases for the test process and restricts the unwanted test glitches.
Due to Artificial Intelligence, a squad of testers can go further than the conventional system of manual testing models for an automated and accuracy-based consistent testing method.
The QA veterans consider the Artificial Intelligence bot as value addition, which can cut down the workload and make their life much better.
Will AI Make Software Testing Irrelevant?
Smart Virtual Assistants such as Alexa, Siri, and Google Home have already shown us how amazing and helpful the world of Artificial Intelligence can be.
You should have come across multiple debates, discussions, as well as conferences around the globe that have messed around with what Artificial Intelligence can and cannot do for humans.
As this technology is so descriptive and comprehensive, there is a lot more to explore and discover about its benefits as well as demerits.
Will the Software Testing AI become efficient enough to ruin the climax predicted by the QA team or it will take a different route?
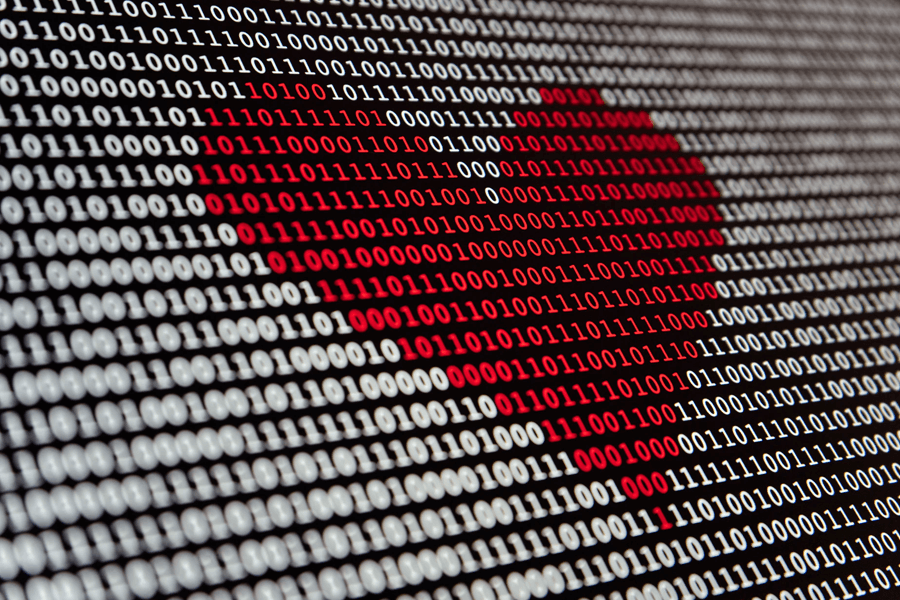
At the moment, AI and ML are the most promising zones when it comes to the quality assurance method.
Every big or small enterprise along with VCs, start-ups, and QA engineers, are investing in multiple ways to ascertain which AI will help in their app development process.
Professionals are focusing on utilizing AI to expedite and automate application testing while keeping Software Testing and QA an integral aspect of it.
This points out the concern, whether artificial intelligence and machine learning can become that much intelligent, will it mar the credibility of QA and testing in the not so distant future?
The VP at Forrester, Diego Lo Giudice has stated that AI will probably help developers and testers be more creative and efficient than making them dysfunctional.
Hence, they should take the benefits of this chance to get an instant ROI. It provides more time for becoming creative and to present more innovation — that can enable them to save their job.
Assuming from this, AI represents itself as a catalyst to the development method instead of a challenger. Nowadays the testing and development are trying to consider AI for different amends as well as advantages.
The cause is that it can have an influence on the structure of the application. You can acquire much with AI that transcends the recurring advantages of automation.
Making sure that your testing methods can be measured and traced is the most crucial concern in this environment. A gaming app requires upgrades and long testing to validate its performance as well as functionality.
In the application of AI-centric tools, the testing process is determined by testers’ goals and the convenience all over the locations. It provides them the chance to adjust and validate and revalidate the ‘gaming’ experience.
Proficiency is the core of perfection, however, measurability and traceability are the major drivers.
There has been a constant evolution in the field of testing and QA all these years. The perfect way to up the ante is by developing a self-learning system rather than a tool-based process.
When an application is being reviewed using an AI-based testing tool every aspect becomes interactive and communicates with one another to make it more intuitive and responsive.
Hence, AI can make testing a ‘self-assessment’ program, where it pushes the unmonitored learning for the app. It is a riveting moment in the testing cycle and app development.
When an AI-based Testing environment will Develop?
An immediate reaction to this query could be, ‘depends on the complexity of your application!’ Test automation has progressed through the development phase to guarantee that testing is still secure and indispensable.
Developing an AI-driven paradigm across software testing guarantees that testing progress will grow with credence and gets quite business-driven.
Artificial Intelligence is going to become more relevant for app development to ensure greater efficiency, recognize defects with convenience and expedite the development as well as testing cycle.
If Test Automation is the part of the game, it can lend a hand to up the ante and make it more intelligent by using data from effective sources.
If the app needs visual confirmation and includes bots, AI comes into the picture to authenticate the performance and also functionalities at different phases.
Through the eyes of developers or businesses with a futuristic approach, you can clearly find AI lending a hand to multiple automated code reviews.
They could also aid in vulnerability evaluations, producing test cases, security checks and even investing in big data.
AI and ML can offer real-time results in terms of the performance of the application.
Although the benefits of AI and ML could be quite a lot, the practical app testing is the liability of testing and QA teams.
Without any planning, test automation can create havoc. Similarly, utilizing ML and AI-based tools without any thought process can be detrimental to delaying your testing process.
Synopsis
The aim is to use the AI-led system to automate the testing and make it work back-end. Such an attempt will allow QA engineers to concentrate on high-priority aspects.
It includes risk and security management, better scalable application, process reassurance, documentation, regulatory cooperation, or monitoring key aspects for the app to keep evolving and providing as predicted.
So the scenario is AI and ML are going to make developers and companies more competent and efficient in terms of testing rather than making them jobless.
All product and company names are trademarks™, registered® or copyright© trademarks of their respective holders. Use of them does not imply any affiliation with or endorsement by them.